THE ECONOMIST: Can Nvidia be dethroned? The start-ups vying for its crown
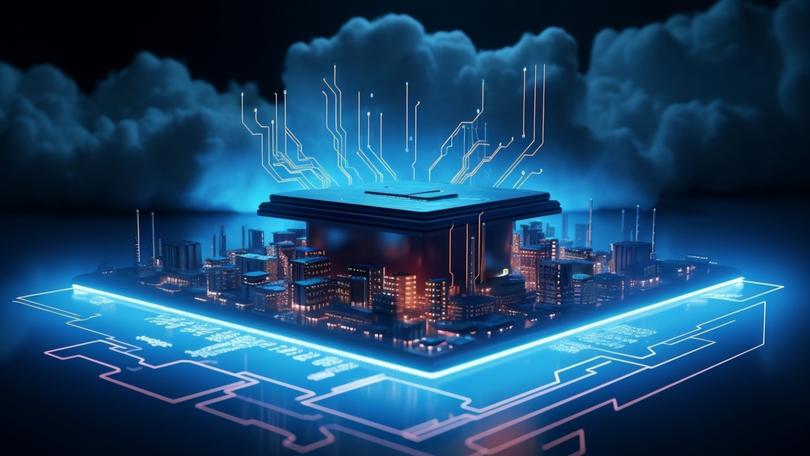
“He who controls the GPUs, controls the universe.”
This spin on a famous line from Dune, a science-fiction classic, is commonly heard these days.
Access to GPUs, and in particular those made by Nvidia, the leading supplier, is vital for any company that wants to be taken seriously in artificial intelligence (AI).
Sign up to The Nightly's newsletters.
Get the first look at the digital newspaper, curated daily stories and breaking headlines delivered to your inbox.
By continuing you agree to our Terms and Privacy Policy.Analysts talk of companies being “GPU-rich” or “GPU-poor”, depending on how many of the chips they have.
Tech bosses boast of their giant stockpiles.
Nvidia’s dominance has driven its market value to more than $2trn. In its latest results, due on May 22nd, it is expected to announce year-on-year revenue growth of more than 200 per cent.
GPUs do the computational heavy-lifting needed to train and operate large ai models.
Yet, oddly, this is not what they were designed for.
The initials stand for “graphics processing unit”, because such chips were originally designed to process video-game graphics.
It turned out that, fortunately for Nvidia, they could be repurposed for AI workloads.
Might it be better to design specialist AI chips from scratch?
That is what many companies, small and large, are now doing in a bid to topple Nvidia.
Dedicated AI chips promise to make building and running AI models faster, cheaper or both. Any firm that can mount a credible threat to the reigning champion will have no shortage of customers, who dislike its lofty prices and limited supplies.
Ordinary processing chips, like those found inside laptop and desktop computers, are in essence designed to do one thing after another.
GPUs, by contrast, contain several thousand processing engines, or “cores”, which let them run thousands of versions of the same simple task (like drawing part of a scene) at the same time. Running AI models similarly involves running lots of copies of the same task in parallel.
Figuring out how to rewrite AI code to run on GPUs was one of the factors that triggered the current AI boom.
Yet GPUs have their limitations, particularly when it comes to the speed with which data can be shuffled on and off them.
Modern AI models run on large numbers of interconnected GPUs and memory chips.
Moving data quickly between them is central to performance.
When training very large AI models, some GPU cores may be idle as much as half of the time as they wait for data.
Andrew Feldman, the boss of Cerebras, a startup based in Sunnyvale, California, likens it to the gridlock in a grocery store on the day before Thanksgiving.
“Everybody’s in a queue, so there are blockages in the parking lot, there are blockages in the aisles, blockages at the checkout. That’s exactly what’s happening with a GPU.”
Cerebras’s response is to put 900,000 cores, plus lots of memory, onto a single, enormous chip, to reduce the complexity of connecting up multiple chips and piping data between them.
Its CS-3 chip is the largest in the world by a factor of 50.
“Our chip is the size of a dinner plate—a GPU is the size of a postage stamp,” says Mr Feldman.
On-chip connections between cores operate hundreds of times faster than connections between separate GPUs, Cerebras claims, while its approach reduces energy consumption by more than half, for a given level of performance, compared with Nvidia’s most powerful GPU offering.
Groq, a startup from Mountain View, California, is taking a different approach.
Its AI chips, called language processing units (LPUs), are optimised to run large language models (LLMs) particularly quickly.
In addition to containing their own memory, these chips also act as routers, passing data among the interconnected LPUs.
Clever routing software eliminates the variation in latency, or time spent waiting for data, allowing the whole system to run in lockstep.
This greatly boosts efficiency, and thus speed: Groq says its lpu can run big LLMs ten times faster than existing systems.
Yet another approach is that taken by MatX, also based in California.
Software is king,
GPUs contain features and circuitry that provide flexibility for graphics, but are not needed for LLMs, says Reiner Pope, one of the firm’s co-founders.
The GPU-like chip his firm is working on gets rid of such unnecessary cruft, boosting performance by doing fewer things better.
Other startups in this area include Hailo, based in Israel, which raised $120m in funding in April; Taalas, based in Toronto; Tenstorrent, an American firm using the open-source risc v architecture to build AI chips; and Graphcore, a British company that was early to the game but failed to gain traction, and is thought to be about to sell itself to SoftBank, a Japanese conglomerate. Big tech firms are also building their own AI chips.
Google has developed its own “tensor processing units” (TPUs), which it makes available as a cloud-computing service. (It unveiled the latest version of the TPU, known as Trillium, on May 14th.) Amazon, Meta and Microsoft have also made custom chips for cloud-based AI; Openai is planning to do so.
And AMD and Intel, two big incumbent chipmakers, make GPU-like chips in an effort to compete with Nvidia.
Is greatness a transitory experience?
One danger for the newcomers is that their efforts at specialisation could go too far. Designing a chip typically takes two or three years, says Christos Kozyrakis, a computer scientist at Stanford University, which is “a huge amount of time” given how quickly AI models are improving.
The opportunity, he says, is that the startups could end up with a chip that is better at running future models than Nvidia’s less specialised GPUs are. The risk is that they specialise in the wrong thing.
Having previously worked at Google, which developed the currently dominant “transformer” architecture used in LLMs, Mr Pope of MatX is confident that his firm has “a somewhat good crystal ball”.
And if a new approach comes along —“state-space models” are the latest thing — its chip is versatile enough to adapt, he says. Mr Feldman says all modern AI is still just “sparse linear algebra” under the hood, which Cerebras’s chip can do very quickly.
Another challenge is that Nvidia’s software layer for programming its GPUs, known as cuda, is a de facto industry standard, despite being notoriously fiddly to use.
“Software is king,” says Mr Kozyrakis of Stanford, and Nvidia has a significant advantage, having built up its software ecosystem over many years.
AI-chip startups will succeed only if they can persuade programmers to rejig their code to run on their new chips.
They offer software toolkits to do this, and provide compatibility with the major machine-learning frameworks.
But tweaking software to optimise performance on a new architecture is a difficult and complex business — yet another reason Nvidia is hard to dislodge.
The biggest customers for AI chips, and the systems built around them, include model-builders (such as Openai, Anthropic and Mistral) and tech giants (such as Amazon, Meta, Microsoft and Google).
It may make sense for such companies to acquire an AI-chip startup, and keep its technology to themselves, in the hope of besting the competition. Instead of trying to compete with Nvidia, chip startups could position themselves as acquisition targets.
Mr Pope says MatX is targeting the “top tier” of the market, which suggests that it hopes to sell its chips — if not the whole company — to the likes of Openai, Google or Anthropic, whose AI models are the most advanced.
“We would be happy with many kinds of exit,” he says, “but we think there is a sustainable business here as a standalone company.”
That remains to be seen. Cerebras, for its part, is said to be preparing for an initial public offering.
So far none of the startups has made even a small dent in Nvidia’s dominant position. But there are plenty of people hoping that one of them will.